The Ethics of Artificial Intelligence in Decision-Making for Social Programs
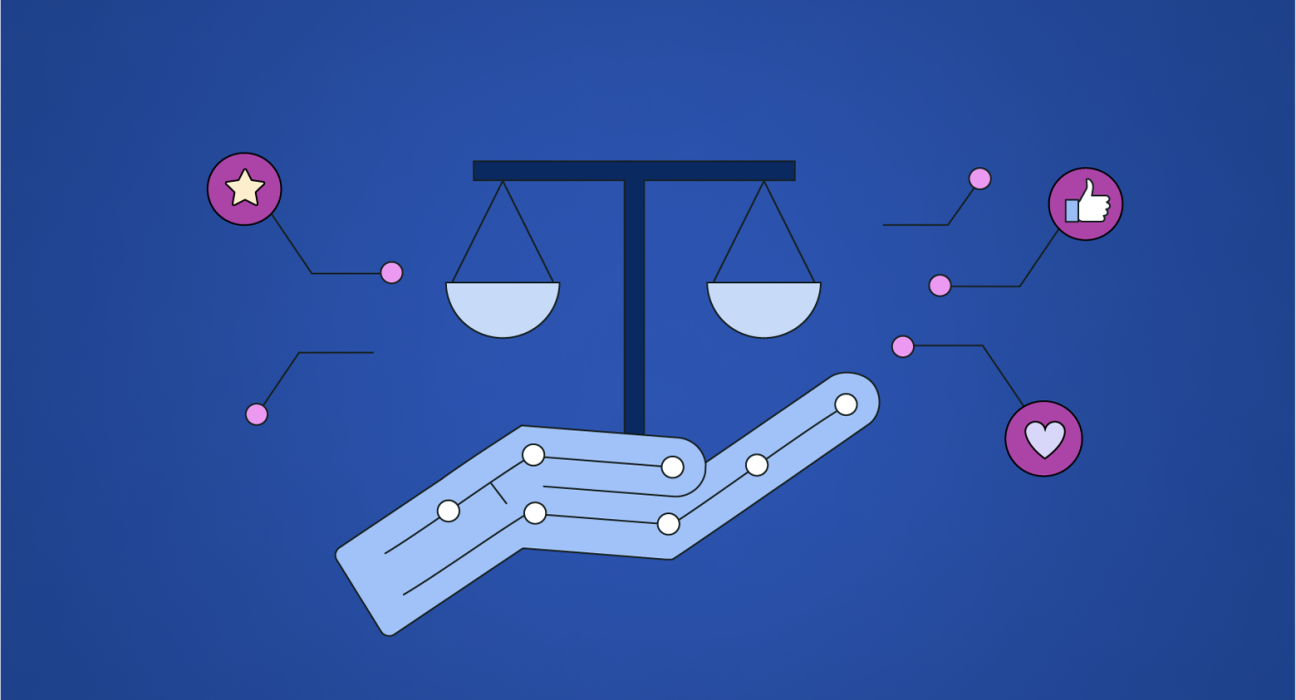
The integration of artificial intelligence (AI) into decision-making for social programs has the potential to revolutionize how resources are allocated, needs are assessed, and outcomes are achieved. However, the use of AI in this sensitive domain raises significant ethical concerns. Balancing efficiency and fairness, ensuring transparency, and maintaining accountability are critical challenges that must be addressed to use AI responsibly in social programs.
AI in Social Programs: Opportunities and Risks
AI systems are increasingly being deployed to support social initiatives, such as welfare distribution, healthcare allocation, and educational resource planning. These technologies offer several advantages:
- Efficiency: Automating data analysis can streamline processes, reducing delays and administrative costs.
- Personalization: AI algorithms can tailor interventions to individual needs, maximizing program effectiveness.
- Scalability: AI enables large-scale operations that are otherwise resource-intensive.
However, the risks are equally profound:
- Bias and Discrimination: AI systems can perpetuate or even amplify biases present in the training data, leading to unfair treatment of marginalized groups.
- Opacity: Complex algorithms often function as “black boxes,” making it difficult to understand or challenge their decisions.
- Accountability Gaps: Determining responsibility for errors or harm caused by AI systems is a persistent ethical dilemma.
Key Ethical Concerns
1. Fairness and Equity
AI systems must ensure that decisions are free from bias and do not disproportionately harm vulnerable populations. For example:
- Training Data Bias: Historical data used to train AI systems may reflect existing societal inequalities, perpetuating them in new decisions.
- Algorithmic Disparities: Even well-designed algorithms can inadvertently prioritize certain groups over others, exacerbating inequities.
2. Transparency and Explainability
Stakeholders must understand how AI systems make decisions, particularly in critical areas like housing allocation or healthcare prioritization.
- Challenges: Complex machine learning models, such as neural networks, are often difficult to interpret.
- Solutions: Developing explainable AI techniques can make systems more transparent and decisions more understandable.
3. Accountability
Who is responsible when an AI system makes an unfair or harmful decision?
- Blurred Lines: Responsibility can be diffused among developers, operators, and users.
- Ethical Frameworks: Clear guidelines and accountability structures must be established to address potential harms.
4. Privacy and Consent
AI systems often rely on vast amounts of personal data, raising privacy concerns.
- Ethical Use of Data: Programs must ensure that data collection and usage comply with privacy laws and respect individual consent.
Case Studies
- Healthcare Allocation: AI-driven tools used during the COVID-19 pandemic to allocate ventilators and vaccines faced criticism for prioritizing certain demographics over others, highlighting fairness concerns.
- Welfare Programs: AI systems deployed in welfare fraud detection have wrongly flagged individuals, resulting in unjust penalties.
- Predictive Policing: In some jurisdictions, AI tools have been used to predict areas or individuals likely to commit crimes, raising questions about profiling and systemic bias.
Ethical Frameworks for AI in Social Programs
To address these challenges, several strategies can guide ethical AI use:
1. Inclusive Design
Engage diverse stakeholders, including affected communities, in the development and deployment of AI systems to ensure fairness and representation.
2. Auditing and Monitoring
Regular audits of AI systems can identify and mitigate biases, ensuring equitable outcomes over time.
3. Transparency Standards
Develop and enforce standards for explainable AI to make decision-making processes clear and accessible.
4. Legal and Ethical Oversight
Establish regulatory bodies to oversee AI deployment in social programs, ensuring compliance with ethical and legal standards.
5. Public Awareness and Education
Equip individuals with the knowledge to understand and question AI-driven decisions, empowering them to advocate for ethical practices.
Conclusion
The use of artificial intelligence in decision-making for social programs offers transformative potential but demands a rigorous ethical approach. By addressing issues of fairness, transparency, accountability, and privacy, society can harness AI to promote equity and justice while minimizing harm. A collaborative effort between technologists, policymakers, and communities is essential to ensure that AI serves the greater good in social programs.