Addressing Systemic Bias in Algorithms and Big Data
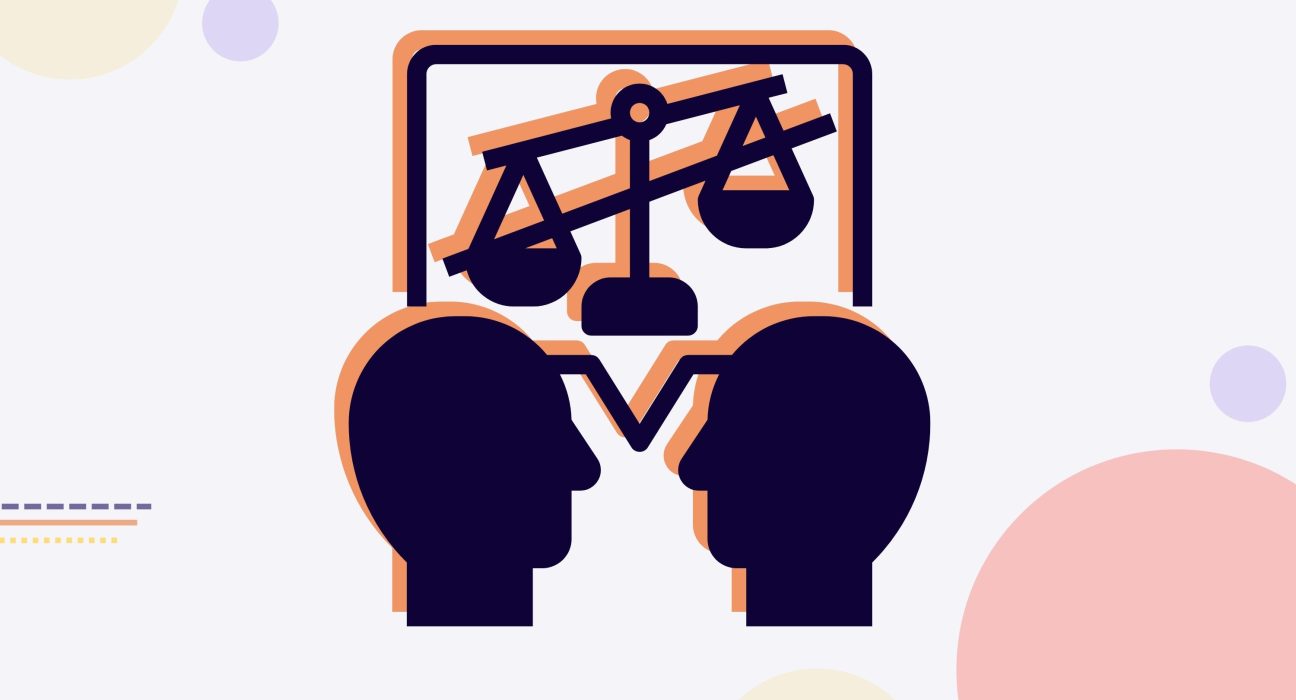
Systemic bias in algorithms and big data has become a critical issue as technology continues to influence decision-making processes. This article explores the origins of bias in algorithms, the consequences of biased data, and the steps needed to ensure fairness and accountability in AI and data systems.
Addressing Systemic Bias in Algorithms and Big Data
As artificial intelligence (AI) and big data continue to shape various aspects of modern life—from hiring practices and loan approvals to criminal justice and healthcare—concerns about systemic bias in algorithms have grown. While algorithms are often seen as impartial and objective, they can perpetuate and even exacerbate biases present in the data they are trained on. Understanding and addressing systemic bias in algorithms and big data is crucial to ensuring fairness, equality, and accountability in the digital age.
The Origins of Systemic Bias in Algorithms
Systemic bias in algorithms arises when the data used to train machine learning models reflects historical inequalities, stereotypes, or discriminatory practices. Data sets often include real-world examples that mirror existing social biases, whether related to race, gender, socioeconomic status, or other factors. For example, an algorithm trained on historical hiring data may inadvertently favor candidates from a specific demographic group, reinforcing patterns of exclusion.
In many cases, bias enters algorithms through the design and development stages, where human decisions about what data to collect and how to interpret it play a significant role. Developers may unintentionally introduce bias based on their own assumptions, leading to algorithms that disadvantage certain groups. Additionally, biased data can result from structural inequalities in society, where certain populations are underrepresented or misrepresented in the data used to train algorithms.
Consequences of Biased Algorithms
The consequences of biased algorithms can be far-reaching, with negative effects on individuals and entire communities. When algorithms are used in decision-making processes, such as hiring, lending, or law enforcement, biased outcomes can reinforce discrimination and perpetuate societal inequalities. For example, an algorithm used by a hiring platform that has been trained on biased data may prioritize candidates of a certain race or gender, leading to a lack of diversity in the workforce. Similarly, biased predictive policing algorithms can disproportionately target minority communities, exacerbating existing disparities in the criminal justice system.
In the healthcare sector, biased algorithms may lead to unequal access to treatment or misdiagnosis, particularly for underrepresented racial or ethnic groups. This can result in worse health outcomes for certain populations and contribute to health inequities.
Types of Bias in Big Data and Algorithms
Bias in algorithms and big data can take several forms, each of which requires different strategies for mitigation:
- Historical Bias: This type of bias arises from past social inequalities or discrimination that are reflected in historical data. For instance, if an algorithm is trained on data from a healthcare system that has historically neglected certain racial or ethnic groups, it may produce biased recommendations or treatment plans.
- Sampling Bias: Sampling bias occurs when the data used to train an algorithm is not representative of the broader population. If certain groups are underrepresented or overrepresented in the data, the resulting algorithm may fail to accurately predict outcomes for those groups.
- Measurement Bias: Measurement bias occurs when the data collected is inaccurate or inconsistent. For example, if data collection methods are flawed or if certain variables are incorrectly measured, the resulting algorithm may make incorrect predictions.
- Prejudicial Bias: Prejudicial bias happens when the values or assumptions of the developers influence the design and operation of an algorithm. This type of bias is often subtle but can lead to algorithms that reflect societal prejudices, such as stereotypes about gender roles or racial profiling.
The Role of Data Collection and Representation
To address systemic bias in algorithms, it is essential to focus on the data collection process. Ensuring that data is representative of diverse populations and experiences is a key step in mitigating bias. This includes collecting data from underrepresented groups and ensuring that the data accurately reflects the diversity of the real world.
Developers must also be mindful of the limitations of the data they are using. If data collection methods are flawed or biased, this will translate into biased algorithms. Additionally, it is important to consider the social and cultural contexts in which the data is collected to ensure that it captures a comprehensive view of the issues at hand.
Solutions for Addressing Bias in Algorithms
There are several strategies that can help reduce systemic bias in algorithms and big data systems, including:
- Diverse Data Sets: One of the most effective ways to reduce bias is by ensuring that data sets are diverse and representative of different demographic groups. This includes collecting data from marginalized or historically underrepresented communities to prevent the exclusion of key perspectives.
- Bias Audits and Testing: Regular bias audits and testing of algorithms can help identify and address potential sources of bias. By running algorithms through a variety of scenarios and examining the outcomes for different groups, developers can identify patterns of discrimination and take corrective action.
- Transparency and Accountability: Increasing transparency in the development and deployment of algorithms is crucial for addressing bias. This involves making the algorithms and data sets used publicly available for scrutiny and allowing independent researchers and organizations to assess their fairness.
- Fairness Metrics: Implementing fairness metrics in algorithmic design can help quantify bias and ensure that algorithms are meeting equity goals. These metrics allow developers to assess whether their algorithms produce equitable outcomes for all groups and adjust them accordingly.
- Human Oversight: While automation and AI play a crucial role in modern decision-making, human oversight remains essential for addressing bias. Developers should ensure that algorithms are regularly reviewed and that there is a process for intervening if biased outcomes are identified.
- Inclusive Design and Development: Ensuring that diverse teams of developers, data scientists, and researchers are involved in the design and development of algorithms is key to minimizing bias. Diverse teams bring different perspectives and are more likely to recognize potential sources of bias that others may overlook.
The Importance of Ethical AI
As the role of AI and algorithms continues to expand in various sectors, the need for ethical AI becomes increasingly important. Ethical AI emphasizes the creation of systems that promote fairness, accountability, and transparency while minimizing harm. Addressing systemic bias is a core component of ethical AI, as biased algorithms can perpetuate discrimination and harm vulnerable populations.
To achieve ethical AI, developers must prioritize fairness in the design and deployment of algorithms, ensuring that they do not disproportionately harm or disadvantage certain groups. This requires a commitment to continuous improvement and the willingness to adapt systems as new biases are identified.
Conclusion
Addressing systemic bias in algorithms and big data is essential for ensuring that technology serves all people equitably. By focusing on diverse data collection, transparency, fairness metrics, and ethical AI practices, developers can mitigate the risks of bias and create algorithms that promote justice and inclusion. As society continues to integrate AI into everyday decision-making, it is crucial that we remain vigilant about the potential for bias and work collectively to build systems that reflect our shared values of fairness, equality, and accountability.